The growing focus on artificial intelligence has become a factor for all parts of the economy, including the packaging sector.
Ayman Shoukry, chief technology officer at cloud-based specification data platform Specright, recently wrote a piece outlining the possibilities and pitfalls of growing AI adoption. Shoukry’s career includes 15 years as a developer at Microsoft, along with stints at companies such as HireRight and Amazon, which gives him unique insight into adapting broader technology trends for packaging applications.
Packaging Dive recently spoke with Shoukry about how establishing standardized data systems is foundational for any packaging company looking to take advantage of AI in their business. This has already become a growing factor as more companies are asked to provide data for extended producer responsibility systems, emissions accounting and other evolving areas.
This interview has been edited for length and clarity.
PACKAGING DIVE: AI itself is not new, but a lot of the hype is new this year. What feels different to you about the AI discussion for packaging this year?
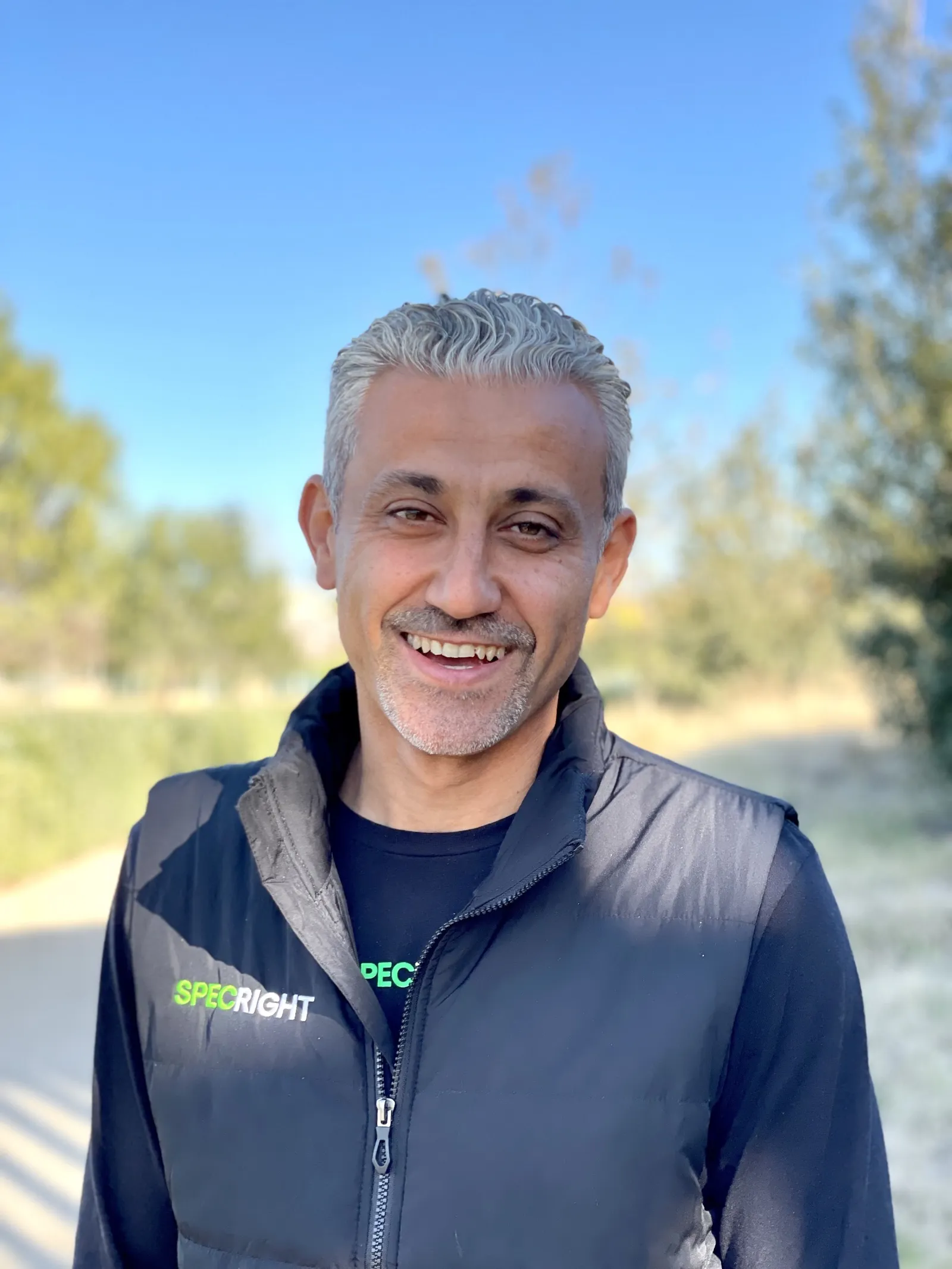
AYMAN SHOUKRY: AI and machine learning have existed for a long time now. It's now becoming more of something the consumer can get their hands on, which is what's happened in ChatGPT and others. Then everybody started to take a look at it, including packaging engineers.
We do have a platform that includes all the specifications — product specifications, specification management — which includes packaging, quality, new product development and all those areas. But the interesting part is questions coming from our customers: “Hey Ayman, what do we do with ChatGPT for packaging?"
There's tons of scenarios that we can enable, whether that's new product development, whether it's things even like [regulation]. I can say to ChatGPT: “Hey, here's my product specifications, here's the vendors that I'm dealing with, my suppliers. Am I impacted with anything that's happening? Am I going to be delayed in my sourcing? Am I going to be delayed in my production, manufacturing and all that kind of stuff which affects packaging?” Those are the types of questions people are starting to ask.
Yes, we can start asking all of those questions, but do you have the right data to feed the models? ... All these predictions are as good as your data. Yes, it's enabling new scenarios. But let's be honest, it's a garbage in, garbage out kind of thing. If you have bad data in, you're going to have bad data out.
You’ve been out telling the industry for years that they need better data, and people largely know that, but it’s not done yet. Your piece for Forbes mentioned regulatory and tax pressures as drivers moving that along. Would it be fair to think of AI as yet another motivating factor for companies to clean up their data?
Absolutely. So we've been getting some customers asking us to take advantage of AI and machine learning and all that stuff and our answer is get the data in and then they can enable those advanced scenarios. If you look at EPR reporting — and Europe is more advanced than here — you're going to get fined if you don't have your data together. So that is one way it's forcing [companies] to get the right data.
It is harder, though, because unfortunately it's not always your data only in the supply chain world. I mentioned that in the article as well as an example about carbon footprint — your [Scope 1, 2 and 3 greenhouse gas emissions]. Most people's carbon footprint is coming through your Scope 3, which is basically that footprint of your suppliers and their suppliers. So it's harder to get all this data in one place and start even knowing all of that and reporting on it.
And that's why we always say supply chain is going to be a collaborative of AI and machine learning. It has to be network-based and not just one-company-based. You have to be able to share this information about your products, about your suppliers — and everybody has access to that in a networked [system] — so that you can start reporting and say, “Okay, I depend on supplier A, B, and C.” And if everyone starts reporting their own Scope 1 and 2, then their 1 and 2 is my 3. Then you start being able to run a lifecycle assessment that measures in a click of a button, versus spending weeks and weeks collecting data to run the LCA.
I'm a developer by heart. It reminds me of the open source world of code. Like in the old days, it used to be my code is on my machine. And it's my IP, and I keep everything there and that's the most important part. Now I know the innovation I'm getting from just open sourcing ... is a lot better. And I think we are at an inflection point in the supply chain world where this is eventually going to happen.
Everything that you put in your product specs you can probably, given the right time, reverse engineer it. You can reverse engineer what's inside: the ingredients and the formulas, how you're packaging it, the different packaging formulas. It's going to become more of a collaboration where everybody publishes that.
Being able to have that network, the open source of your specs, that's going to be a key that transforms our industry in general.
Data flows through everything, but are there any other key categories in the packaging sector that you think are going to be affected by AI or have trouble transitioning? For example, there's a lot of legacy machinery, a lot of older operating systems.
I think the major challenges are going to be some of the older systems — whether hardware or software systems — especially the older homegrown ones. Most of the bigger companies — and you'd be surprised how big they are, they've been in existence a long time — when they started they were ahead, and they built their own systems. But those homegrown systems, they're not their core business. Their core business is the final product at the end. So they've never innovated through the years because it's working.
And now they've discovered they need to get much more data from those homegrown systems. They need to be able to link all the subsystems together. If I have a vendor in some specific area that I depend on, and there's a shortage, I need to be able to link it all the way to what manufacturing lines will be impacted so that they can shift it when something happens instantly.
Those homegrown systems — sometimes it's Excel sheets and PDFs that you're tracking stuff in — are not going to scale if you want to enable those scenarios. Because at that moment, that's where some of the predictive analytics and machine learning will kick in and say, “Okay, you just had a machine part that fell down. You know that you bought those five machines at the same time. So you probably expect the same parts in all the five.” When you order the parts, it's probably good to order five parts, which will cost you less because now you're bulk ordering, versus two. Because in less than six months from now you’re probably going to have the same three, four machines that are going to need the same parts.
But again, you have to know that information, start linking that together all of the way. It's not easy. And we know that and we've seen it. The majority of our bigger customers, believe it or not, are not equipped yet. Their suppliers know more about their products than themselves because they've been with the same supplier forever.
Another example you used was how the industry coalesced around the process for certificates of analysis and insurance. What lessons from that can be applied to this moment, or what things that didn't go well could be better this time?
It's basically the standards. The only way you used to be able to say, “Hey, is the insurance or analysis expired or not?” used to be having thousands of documents, PDFs sent your way. And you're opening one by one to see did the insurance expire or not for that supplier. Sometimes you catch it, sometimes you don't. Sometimes even you catch it afterwards and you start pinging the supplier.
We were able to — it's not just we as Specright, but others as well in the industry — do intelligent document processing where you can feed our models those tens of thousands of documents. We'll be able to digitize those into actual data fields that you can report on. It gets uploaded monthly and the system is able to say, “Did you miss that or not?” And it feeds the model to improve the next time you process another document or another COA or COI. And at that moment you have your data digitized, you even get monthly reports and it even pings the supplier: “Hey can you upload your new certificate of insurance or certificate of analysis?”
Intelligent document processing is not rocket science, people have done it before. And it's a similar model we have to learn from. Supply chain is a lot different across the board. It’s not going to be exactly 100% the same, but at least closer from formats and templates. That's where you’re going to start enabling even better machine learning and better AI, and honestly even better just reporting in general.
How are you using AI in your own business? What does all this mean for Specright?
We're looking at different models. One is predictive analytics, of quality and other areas in the platform, as well as intelligent document processing which we do for COAs and COIs. For our own business we are looking as well for different ways of optimizing our own customer access. So it [could apply to] even our own sales strategies and other areas as well.
Another part is our support. Do we start even using tools like ChatGPT and others for our customer support? That's something we haven’t yet started, but definitely something that we're starting to think about.
There’s no way to predict where this is all going, but there are a lot of questions about how AI gets regulated or whether it speeds up or slows down. If customers are asking you what to watch for in the next six months, what do you tell them?
I honestly tell them there's going to be lots of models out there, innovative models. Whether it's for packaging or general supply chain, there are going to be multiple companies that go into more vertical models. Like healthcare AI, supply chain AI. It's going to be manufacturing only, it's going to be packaging only. There are going to be multiple verticals. And the only way you're going to take advantage of those different models is to get your data right first.
Especially in the packaging industry, people are used to doing lots of stuff manually right now. You'd be surprised by the amount of yellow sticky notes that we're still using in some industries. For some people it's working. Yes, it could get better and better. But if you get it wrong, your confidence in the models is going to be [affected]. The hardest part is to start getting that confidence back.
So it's that inflection point. You will see people that — again in my opinion, I could be wrong — completely adopt everything and be on the verge of the right machine learning, the AI innovations, because they got the data right initially. And there are going to be others that think they can take advantage of it, but they start applying it not to the right data; and at that moment, they're going to lose confidence and they're not going to take advantage of any of those, and they're going to be left behind.